Program
All times GMT+2.
SYNT 2023 | ||
---|---|---|
09:00 - 09:10 |
Welcome
|
|
Session 1 | ||
Session Chair: Bettina Könighofer | ||
09:10 - 10:10 |
Invited Talk: Intelligent and Dependable Decision-Making Under Uncertainty Nils Jansen, Radboud University Nijmegen |
|
10:10 - 10:30 |
Reactive Synthesis of Linear Temporal Logic on Finite Traces Shufang Zhu |
|
10:30 - 11:00 | Break |
|
Session 2 | ||
Session Chair: Anna Lukina | ||
11:00 - 11:20 |
Taming Large Bounds in Synthesis from Bounded-Liveness Specifications (Extended Abstract) Philippe Heim and Rayna Dimitrova |
|
11:20 - 11:40 |
Easy-to-Use Shield Synthesis for RL with Tempest Stefan Pranger |
|
11:40 - 12:00 |
Shielded Reinforcement Learning for Hybrid Systems Asger Horn Brorholt, Peter Gjøl Jensen, Kim Guldstrand Larsen, Florian Lorber and Christian Schilling |
|
12:00 - 14:10 | Lunch |
|
Session 3 | ||
Session Chair: Swen Jacobs | ||
14:10 - 15:10 |
Invited Talk: Making New Friends in Software Synthesis Ruzica Piskac, Yale University |
|
15:10 - 15:30 |
Reinforcement Learning for Syntax-Guided Synthesis Julian Parsert and Elizabeth Polgreen |
|
15:30 - 16:00 | Break |
|
Session 4 | ||
Session Chair: Bettina Könighofer | ||
16:00 - 16:20 |
On-the-fly reactive synthesis modulo theories (Extended abstract) Andoni Rodriguez and César Sánchez |
|
16:20 - 16:40 |
Genetic Algorithms for Searching a Matrix of Metagrammars for Synthesis Yixuan Li, Federico Mora, Elizabeth Polgreen and Sanjit A. Seshia |
|
16:40 - 17:00 |
NeuroSynt: A Neuro-symbolic Portfolio Solver for Reactive Synthesis Matthias Cosler, Bernd Finkbeiner, Christopher Hahn, Ayham Omar and Frederik Schmitt |
|
17:00 - 17:20 |
SYNTCOMP 2023 Results Swen Jacobs and Guillermo Alberto Perez |
Invited Speakers
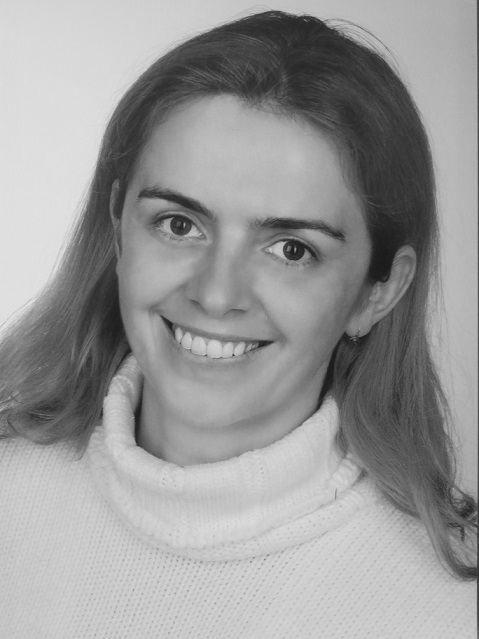
- Bio: Ruzica Piskac is an associate professor of computer science at Yale University. Her research interests span the areas of programming languages, software verification, automated reasoning, and code synthesis. A common thread in Ruzica's research is improving software reliability and trustworthiness using formal techniques. Ruzica joined Yale in 2013 as an assistant professor and prior to that, she was an independent research group leader at the Max Planck Institute for Software Systems in Germany. In July 2019, she was named the Donna L. Dubinsky Associate Professor of Computer Science, one of the highest recognition that an untenured faculty member at Yale can receive. Ruzica has received various recognitions for research and teaching, including the Patrick Denantes Prize for her PhD thesis, a CACM Research Highlight paper, an NSF CAREER award, the Facebook Communications and Networking award, the Microsoft Research Award for the Software Engineering Innovation Foundation (SEIF), the Amazon Research Award, and the 2019 Ackerman Award for Teaching and Mentoring.
- Title: Making New Friends in Software Synthesis
-
Abstract: While reactive synthesis and syntax-guided synthesis (SyGuS) have seen enormous progress in recent years, combining the two approaches has remained a challenge. To overcome this obstacle, we introduced Temporal Stream Logic modulo theories (TSL)L, a new temporal logic that separates control and data. We developed a CEGAR-like synthesis approach for the construction of implementations that are guaranteed to satisfy a TSL specification for all possible instantiations of the data processing functions. However, specifications often involve interpreted functions: for example, arithmetic functions or string manipulations. We extended TSL to Temporal Stream Logic modulo theories (TSL-MT), a framework that unites the two approaches to synthesize a single
program. In our approach, reactive synthesis and SyGuS collaborate in the synthesis process, and generate executable code that implements both reactive and data-level properties. We demonstrate the applicability of our approach over a set of real-world benchmarks.
This is a joint work with Wonhyuk Choi, Bernd Finkbeiner, Felix Klein, and Mark Santolucito.
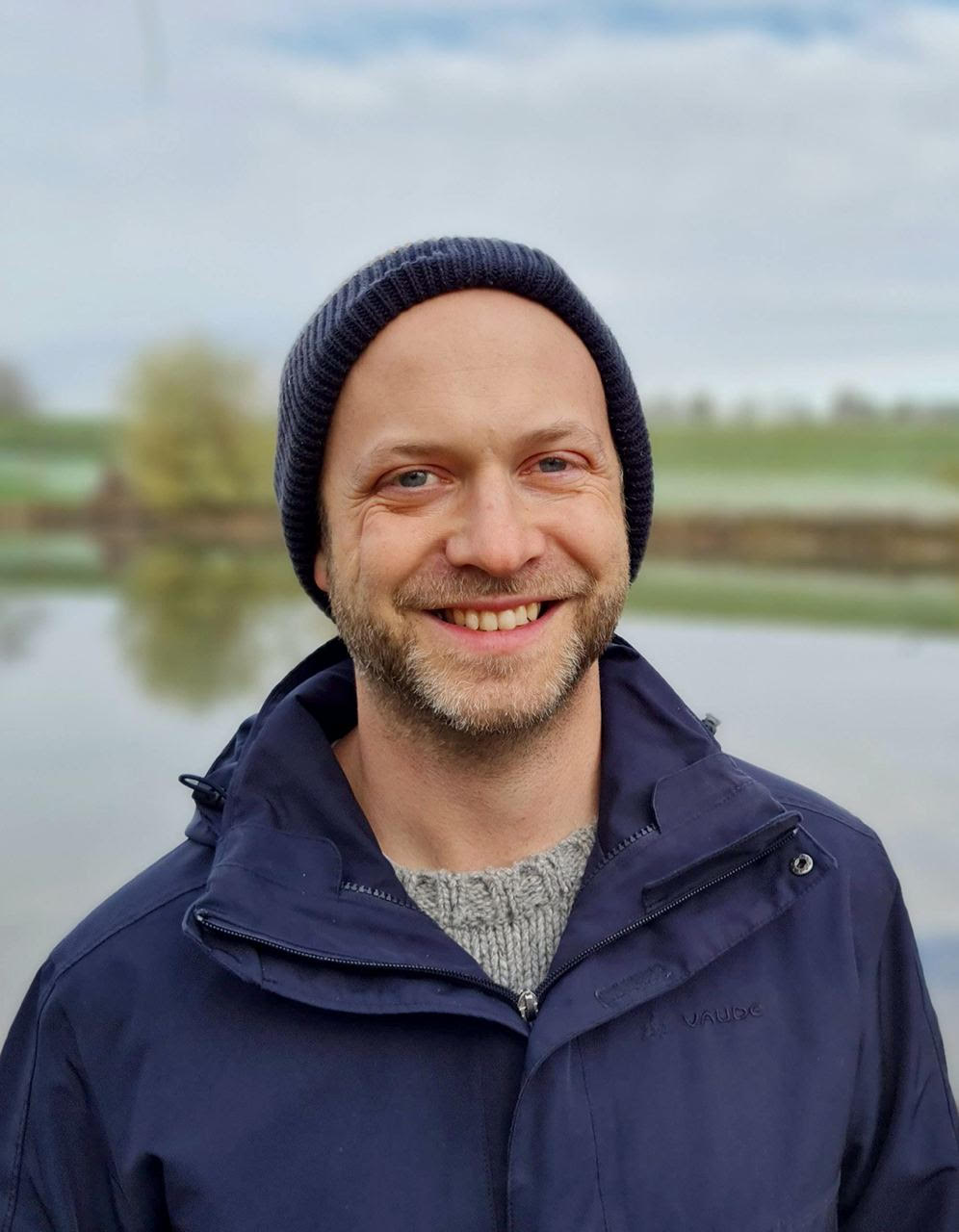
- Bio: Nils Jansen is an associate professor with the Institute for Computing and Information Science (ICIS) at Radboud University, Nijmegen, The Netherlands. He received his Ph.D. with distinction from RWTH Aachen University, Germany, in 2015. Before Radboud University, he was a research associate at the University of Texas at Austin. His research is on intelligent decision-making under uncertainty, focusing on formal reasoning about the safety and dependability of artificial intelligence (AI). He holds several grants in academic and industrial settings, including an ERC starting grant with the title: Data-Driven Verification and Learning Under Uncertainty (DEUCE).
- Title: Intelligent and Dependable Decision-Making Under Uncertainty
- Abstract: This talk highlights our vision of foundational and application-driven research toward safety and dependability in artificial intelligence (AI). We take a broad stance on AI that combines formal methods, machine learning, and control theory. As part of this research line, we study problems inspired by autonomous systems, planning in robotics, and industrial applications. We consider reinforcement learning (RL) as a specific machine learning technique for decision-making under uncertainty. RL generally learns to behave optimally via trial and error. Consequently, and despite its massive success in the past years, RL lacks mechanisms to ensure safe and correct behavior. Formal methods, in particular formal verification, is a research area that provides formal guarantees of a system’s correctness and safety based on rigorous methods and precise specifications. Yet, fundamental challenges have obstructed the effective application of verification to reinforcement learning. Our main objective is to devise novel, data-driven verification methods that tightly integrate with RL. In particular, we develop techniques that address real-world challenges to the safety of AI systems in general: Scalability, expressiveness, and robustness against the uncertainty that occurs when operating in the real world. The overall goal is to advance the real-world deployment of reinforcement learning.